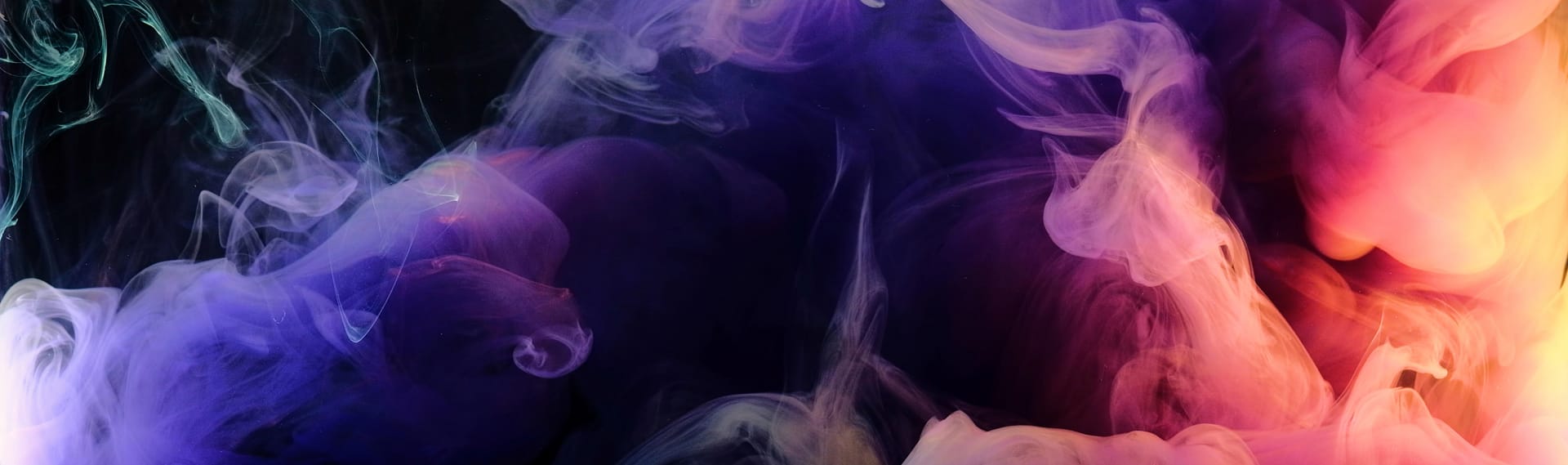
Different Data Models for Different Business Insights

It’s no surprise to anyone that most business’ analytics and decision-making processes have been completely transformed by the data afforded to us by the rapidly changing technological age. That said, this information is obtained and processed by different means, all going into a company’s systems. Have you ever wondered about how these systems are compiled? Typically, that’s when people rely on data models.
Data modelling involves defining the requirements for incoming quantitative information and determining how it will be represented and used within business processes. In other words, without data modelling, organizations and businesses wouldn’t have a centre to build their key operations around.
And so data models are necessary. They have been described as “the basis” of data management and are the core around which companies and organizations can implement important changes in a system or operation — but not all data models are created equal. What you base your data-gathering operations on will require some careful thought and planning.
Developing Data Models
It will be helpful to start with a basic understanding of the different types of data and how they interlap with one another. There are actually 13 types of data, but in 2019 we can focus on three primarily: big data, real-time data, and machine data.
- Big data comes from things like the cloud and people’s use of online and smart systems.
- Real-time data is obtained as market and profit changes occur.
- Machine data, which involves both big data and real-time data, can lead to machine learning with predictive analysis.
This last point concerning obtaining predictive analyses using machine data is vital to understand and get ahead of your competition. This is because a lot of data models currently lack the ability to transition and adapt with new information. Models in themselves address specific moments in time, but machine learning could change this by way of helping models evolve as they are used, troubleshooting and fixing errors as they are discovered.
This still isn’t relevant, however, if you’re not customizing your organization’s models and considering the different types of data you’re obtaining. Everything is contextual, after all. Build models that will obtain data from the most relevant sources and allow your business or organization’s decision making to be as informed as it can possibly be.

Enlisting New Technologies to Help
With the predictive analysis advancements that have been made through technology, it would be silly not to enlist said technology in your business decision making. Use the new tools available to improve and build your data models.
Especially within the realm of analytics, new technologies are raising the bar for what’s possible in the fields of data collection and informed decision making. As Towards Data Science explained:
These [new technologies] range from the most fundamental techniques, “descriptive analytics”, which involves preparing the data for subsequent analysis, to “predictive analytics” that provide advanced models to forecast and predict future, to the top-notch of analytics called “prescriptive analytics” that utilize machine-based learning algorithms and dynamic rule engines to provide interpretations and recommendations.
One such technology is the cloud. Building cloud-based analytics programs will allow you to pull data from social media, an invaluable resource in 2019. It’s important as well to realize that AI will probably make data models better rather than destroy them, and the more those are implemented in cloud systems and other technology, the better your data models can become. So when new technology is offered to you, take advantage of it — don’t push it away.

Setting Up Safety Nets
What happens if your systems crash? An important part of your data model is your safety nets: business continuity plans (BCPs) and disaster recovery plans (DRPs). BCPs help to keep a business going in the event of a disruption and DRPs are put in place to obtain information lost. Data recovery experts Kroll Ontrack explained the importance of these types of plans(from their experience combatting data loss) as such:
Despite better software solutions and hardware, data loss still occurs … When data is the centre of a business, a loss of frequently used data can sometimes lead to bankruptcy, e.g. when deadlines can´t be met in an ongoing project or databases are not available anymore.
What’s important, however, is that you have these things in place, because no system is perfect. You don’t want to have to start from scratch with a new data model and a system of operations if you don’t have to.
Gaining an understanding of data models comes down to understanding different types of data, to begin with. Furthermore, it’s important to evolve data models and systems with new technology; without it, your analytics won’t improve or account for everything they can. More so, don’t forget your safety net programs like your BCPs and DRPs. In the case of data loss, they will keep your operations moving smoothly and allow you to get back some of what you lost.
Originally posted by Avery Phillips
If you found this article insightful, you might find this blog interesting too.
Follow us on our LinkedIn and Twitter to stay up to date with the latest news in the digital data-driven ecosystem
Digital analytics, optimisation, data science or programmatic expert, and looking for a job?
Check out our latest live vacancies here
Digital agency looking to expand your team with top-tier talent?
Send us your jobs here
Get in contact with us!