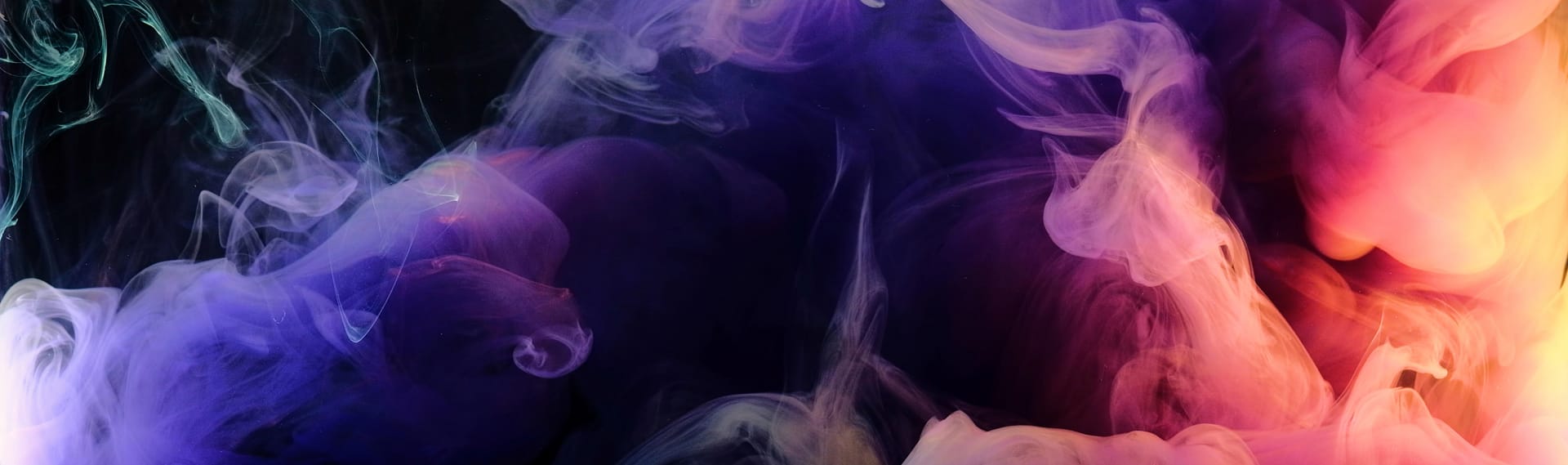
Digital Transformation: 8 Best Practices For Building An Analytics Roadmap

Consider these best practices for making the most of analytics as part of your digital transformation efforts.
The idea of creating an analytics roadmap for the enterprise can be daunting, what with the expansion of new data sources, the proliferation of new analytics systems and tools, and the tremendous demand from the business for faster insights.
But without a roadmap, advanced analytics efforts may underdeliver, increase complexity, or worse. “When organizations don’t have a common vision and roadmap for analytics, each function in the organization tends to establish a partial digital platform to solve for their immediate needs – but in a siloed way – making the overall analytics portfolio ineffective,” says Ashish Verma, managing director and leader of data and analytics modernization at Deloitte Consulting.
“In addition, as data continues to rise in both volume and variety, storage across multiple business functions without capturing value can lead to increasing insight costs without revenue growth,” Verma says.
IT organizations that develop an analytics roadmap create visibility into the transformation required and can better socialize those changes with stakeholders throughout the enterprise, says Verma.
There’s no denying that, as ISG partner Prashant Kelker says, “it is inherently difficult to create a digital transformation and analytics roadmap.” But smart practices are emerging around the process. IT leaders mapping out analytics plans should consider these steps:
1. Involve the business at the outset
By all means, include key stakeholders as early as possible. This will reduce project delays and ensure that all are aligned on the roadmap, says Verma. Understand that you will have to address varying needs and levels of understanding. “Knowledge of AI and analytics will be different for the entire organization,” Kelker says. “The marketing team may be very smart in these areas as they have been doing it for years. But finance or HR, hearing it for the first time, will need significant support to be able to understand and use analytics.”
2. Start with business objectives, not technology
“The biggest challenge we see with organizations going down the analytics journey is that they start with a technology-led approach,” says Jimit Arora, a partner with Everest Group. “For example, companies feel invested to buy the latest big data tools and visualization technologies, and then determine how to create optimal usage.” Those seeking to create an effective analytics strategy should start with key business objectives, such as top-line growth, cost reduction, or risk management.
3. Assess the current state
“Conduct an audit of all existing analytics and data technology in place and how they map to the organization’s analytics needs and goals,” Verma advises. “This [should include] developing a maturity model to measure proficiency across key dimensions and capabilities for the analytics platform.”
Take some time to simplify the sprawl that may have occurred to date. “Legacy applications often incorporate redundant data and processing routines. Involve enterprise and solution architects early to streamline data and processing efficiencies through analytics-enabled architecture,” Verma says.
4. Envision the desired future state
Conduct sessions with key leaders and business partners to prioritize and align on scope to be delivered in the future state to improve acceptance, Verma says. “When creating the vision for the analysis, weigh costs and benefits to the business, alignment to corporate objectives, total cost of ownership, and return on investment to ensure the model delivers value from analytics offerings.”
5. Perform a gap analysis and prioritize
Identify and prioritize the key capabilities that will enable the organization to accelerate its analytics capabilities in a way that will deliver benefits quickly.
“Once the business objectives are in place, enterprises need to make a set of decisions about four distinct building blocks,” Arora says.
Those building blocks are data (both structured and unstructured), tools (technology for data ingestion, analysis, and visualization), analytics and data science talent, and infrastructure (a foundation capable of handling the volume, variety, and velocity of the data and the complexity of the analytics).
6. Build for today, design for tomorrow
Integrating advanced analytics will be an ongoing process, and available technologies and capabilities are evolving quickly. “Design a flexible scalable architecture to support future state analytics workloads [which will include] machine learning and artificial intelligence,” advises Verma.
7. Create a framework for analytics implementations
Develop an end-to-end process for conceiving, developing, and implementing advanced analytics capabilities. “As part of this framework, businesses should consider including focused and measurable activities that can help improve decision-making and drive innovation in products, services, and internal operations through analytics platform modernization,” says Verma. Make sure to socialize and market these best practices.
8. Create a phased approach to analytics transformation
This will “ensure meaningful outputs and allow for agile changes to the business needs,” says Andrew Alpert, managing director with Pace Harmon. Companies that are new to analytics will learn exponentially from the initial deployments to better inform future needs. “Getting these elements right during the first phase is more important than implementing functionality,” Alpert says.
“From there, it will be a journey to establish an optimal data and information model for which information to capture and why, as well as to mature from reports to descriptive analytics and eventually into predictive analytics,” Alpert says. “The architecture can evolve over time as well.”
In addition, within each analytics deployment, IT leaders can mitigate delivery risk by breaking up capabilities into phased releases. “This will provide adequate time and effort for platform stabilization upfront to optimize acceptance risk,” Alpert says.
Originally posted by Stephanie Overby.
If you found this post interesting, you might enjoy reading this blog too.
Follow us on our LinkedIn and Twitter to stay up to date with the latest news in the digital data-driven ecosystem
Digital analytics, optimisation, data science or programmatic expert, and looking for a job?
Check out our latest live vacancies here
Digital agency looking to expand your team with top-tier talent?
Send us your jobs here
Get in contact with us!