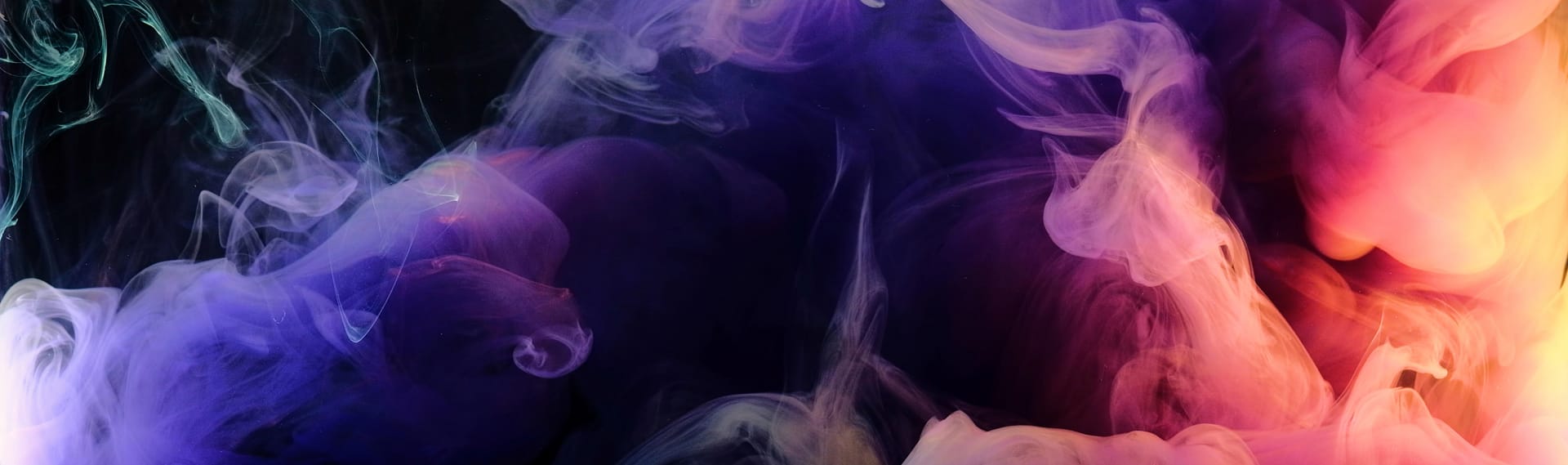
Machine learning maps poverty in Rwanada
It’s impossible to solve a problem if you don’t know what it is. When it comes to sensitive social issues like poverty, data collecting is vital to improving well being and quality of life. However, these situations are often the most difficult to collect data, especially in developing countries where insufficient records or conflict can undermine databases.
Poverty mapping is a detailed way to examine the geographic distribution of poverty and the conditions in which poor people live. Maintaining accurate poverty profiles of a region can be challenging. In some regions, a lack of current records makes it hard to understand present conditions and on-the-ground household surveys can be both expensive and time consuming.
Researchers from the UW Information School and computer science and engineering department are exploring poverty using mobile phone metadata drawn from a database containing billions of interaction records from the largest mobile phone network in Rwanda. Employing machine learning, they were able to estimate an accurate geographic poverty profile of the region.
The research was led by Joshua Blumenstock, who founded the UW Data Science and Analytics Lab and is now an assistant professor at the UC Berkeley School of Information.
Rwanda has made considerable efforts toward poverty reduction, healthcare access, and economic development since the 1994 genocide that occurred there. The number of people living below the poverty line dropped from 78 percent in 1995 to 45 percent in 2011.
This data was supplemented with over 800 phone interviews that recorded data on various wealth indicators for individuals and households. They also analyzed mobile phone histories to determine how patterns in phone usage can be an indicator of an individual’s socioeconomic status using complex machine learning.
Their findings showed that some patterns in phone usage can be reliable indicators of individual wealth. Factors like call frequency, the number of calls made or received, and the number of calls made during business hours gave insight to the habits of people on various ends of the socioeconomic scale.
By applying these algorithms to metadata from 1.5 million Rwandan mobile phone users, researchers were able to estimate the geographic distribution of wealth to a very fine degree. The estimates were almost identical to the Rwandan government’s poverty data from the census from the following year.
The researchers suggested that in developing countries like Rwanda, alternative sources of data collection may prove to be very important. In the full study published in the magazine Science, the researchers confirmed the total cost of the phone survey to be only $12,000 and the total time to administer it as four weeks. Compared to other more direct methods of data collection, this is both cost and time-efficient.
These findings are promising for the future of poverty mapping but also for a variety of other research areas. As machine learning advances, new avenues may open up to study indirect indicators and understand different features of a population. In developed nations, we’ve seen this in the way that analyzing data from social media and the internet can be used to predict electoral outcomes and measure unemployment.
In the future, we could be employing internet usage statistics or satellite imagery to understand vulnerable populations. As we learn more about how machine learning can interact with the social sciences, we can provide a better basis for public policy and aid decisions to best structure interventions that offer relief and more efficiently work to minimize poverty.
Reach writer Rhea John at [email protected]
International digital expert and looking for a job?
Get in contact with us!