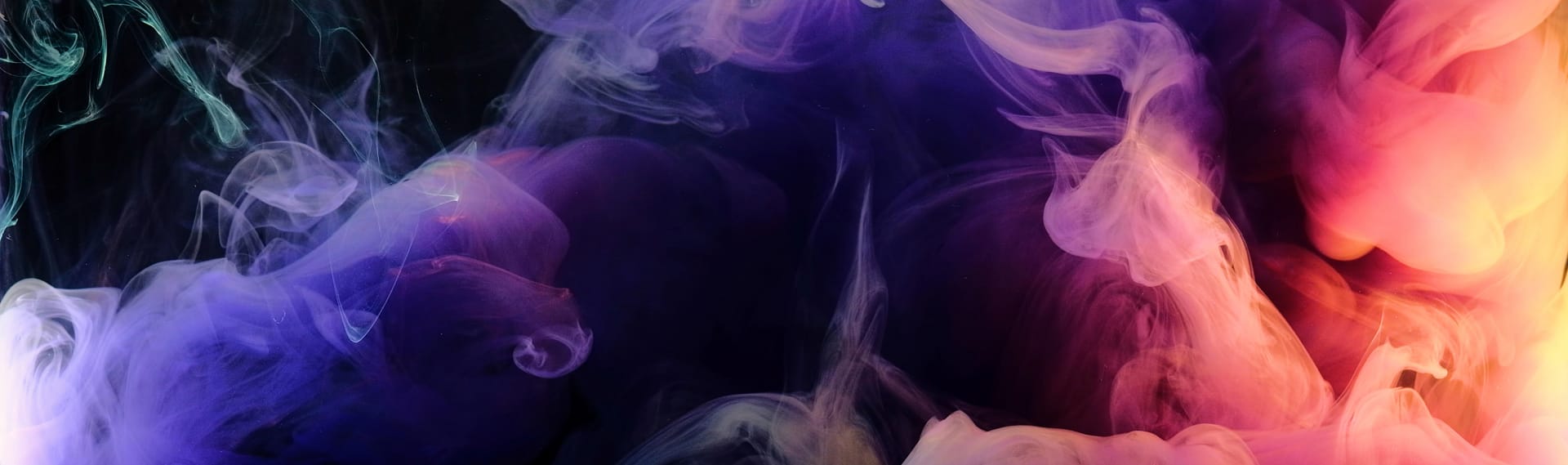
3 Barriers to Effective Data Analytics

Digital information has been growing at such a pace that the term “big data” no longer seems adequate. Perhaps we should be talking about “massive data”, or “gigantic data”.
In any case, the term hides some of the central problems with business analytics today. Many companies have solved or largely solved the problem of how much data there is. Cloud storage makes it easy to store data, and many organisations have made heavy investments in data warehouses to manage their data.
Analytics remains immature
Yet, few have reached a level of maturity with their analytics that can be considered transformational. A global Gartner survey of 196 organisations found that only 9% considered they matched Gartner’s definition for the highest level of maturity, where data and analytics are central to business strategy. Only 44% of respondents in North America and 30% in Europe, the Middle East and Africa considered they were in the top two levels.
So, what is stopping organisations from getting real value from their existing investments in data and analytics? I think there are three key barriers:
- Fragmentation: Data flows from a growing number of sources, including customer transactions, marketing automation systems and the Internet of Things.
This has often resulted in “data islands” across databases and legacy archival systems, with inefficient data duplication and multiple, disconnected repositories of data with inconsistent structures.
Research commissioned by Exasol found that fragmentation is preventing 55% of medium to large organisations from getting full value from their data. To gain a holistic view of business performance, BI solutions and workarounds are sometimes layered on top of those data islands, including the practice of manually pasting data into spreadsheets.
- Performance: Legacy infrastructure has also resulted in inefficiencies and latency in performance as these systems were not built to handle the extreme demands of today’s compute and data-intensive workloads.
Often the net result is that legacy systems get a reputation for not being fit for purpose, especially when getting data into the hands of decision-makers becomes a problematic and lengthy exercise.
- Simplification: To tackle the huge data volumes within the limited constraints of the legacy IT, and the perhaps even more limited resource of the user’s patience, data professionals have often been forced to simplify the data to speed up results. Aggregates, or reports based on an extract of the data, can be generated more quickly and can give a good overview to senior management.
But they don’t give a true understanding of the business or the markets it operates in. Significant anomalies may be averaged out of sight, and it’s difficult to respond meaningfully to a headline figure that isn’t backed with granular detail.
As a result of these barriers, many organisations have BI and analytics solutions that are labour intensive, based on incomplete information, and backward-looking.
Operationalising analytics
These barriers have arisen because organisations have struggled to implement a robust data management philosophy. Data analytics approaches have become as siloed as the data.
The starting point for transformation is to think holistically and take an organisation-wide approach to data strategy and architecture. But there’s no need to start over.
It is possible to build on the existing investments in data warehouses, where you may have huge volumes of historical data and current processes for ingesting new data.
One enabling technology at the forefront of helping organisations modernise and improve the performance and scale of their data infrastructure is an in-memory database.
Processing data in memory helps surface large data sets with fast results, making data professionals’ BI and analytics tools more responsive and interactive.
Memory efficiency also enables businesses to bring together and analyse all the required data, removing the latency and silos that prohibit the transition from BI to data analytics. Meanwhile, an open integration framework ensures organisations can modernise their existing legacy infrastructure piecemeal, without having to rip and replace existing investments.
In-memory databases enable BI reports to be delivered in seconds rather than hours, improving productivity and turning decision makers into true knowledge workers. Businesses can use the new insights they derive to respond to what is happening right now, to drive transformation and to become a data-centric business.
Driving analytics forwards
All business should be aspiring to achieve this immediate, automated view of data. Because when businesses truly master data, they become what Forrester has called “insights-driven business”.
The results speak for themselves. These companies are growing at a rate of 30% annually and could earn $1.8 trillion by 2021.
That’s the real power of data: “insight-driven businesses” don’t just collect data for the sake of it. They use data in a meaningful, insightful way, that creates a very real competitive advantage.
Originally posted by Helena Schwenk.
If you enjoyed reading this article, you might find this post interesting too.
Follow us on our LinkedIn and Twitter to stay up to date with the latest news in the digital data-driven ecosystem
Digital analytics, optimisation, data science or programmatic expert, and looking for a job?
Check out our latest live vacancies here
Digital agency looking to expand your team with top-tier talent?
Send us your jobs here
Get in contact with us!