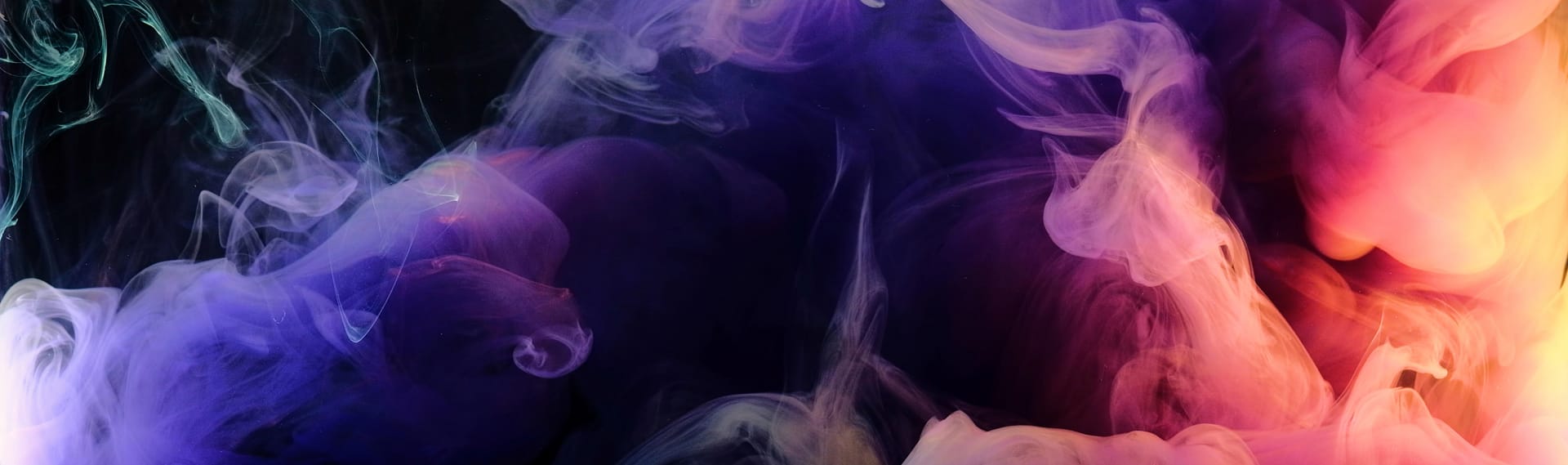
Strengths and limits of analytically driven advice
Strengths and limits of analytically driven advice
Our data allow us to assess what has happened, predict what will happen next and then advise us as to how to respond
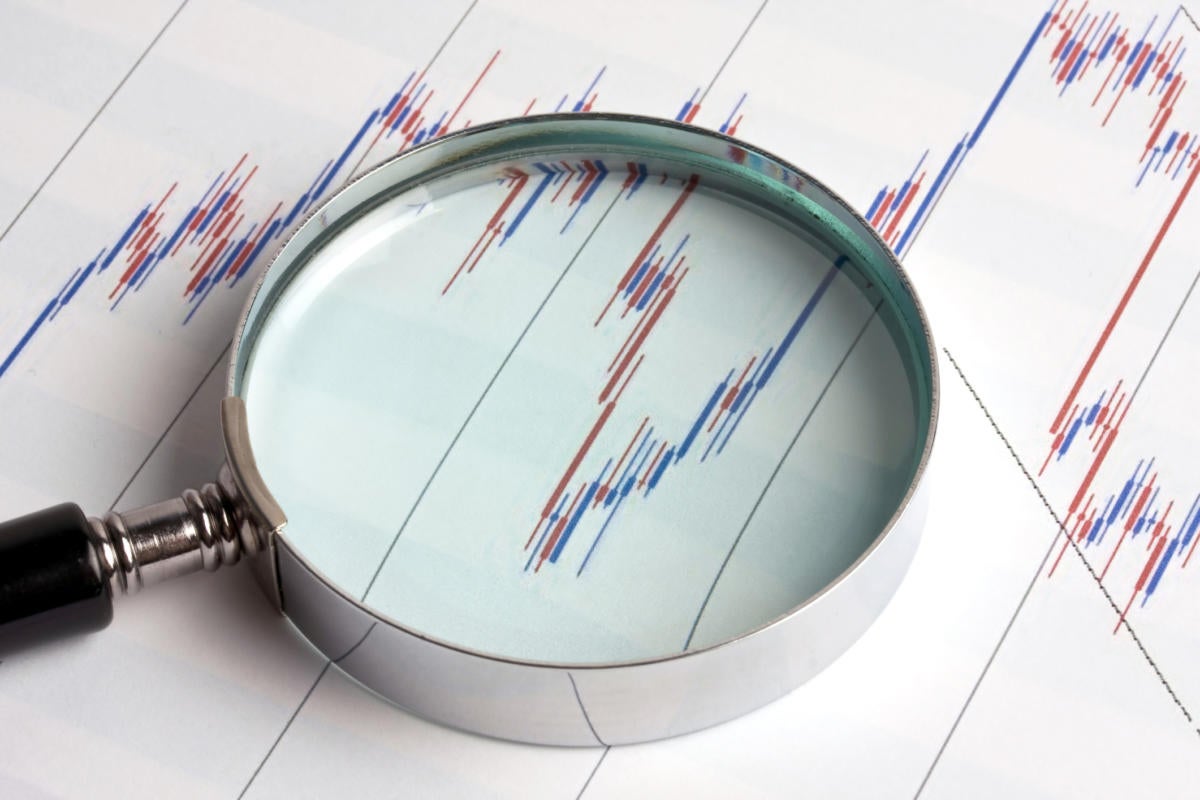
There is no question the data now available to us is incredibly valuable. The data and our ability to analyze it for insight give us a tremendous view into what is happening in the world.
In general, the utility of data analysis breaks down into three main categories: descriptive, predictive, and prescriptive. Somewhat more colloquially, our data allow us to assess what has happened, predict what will happen next, and then advise us as to how to respond.
All three functions depend on the data available and each requires a step up the food chain in terms of data completeness. Even when you have the data required to assess what is happening in the world you might not be able to predict what is going to happen next and even when the data support prediction, they might not support the ability to advise.
Nearly any dataset can be used to provide a picture of what is happening or has happened. The richer the data, the richer the description. To predict, however, the dataset has to include historical information about the features that you want to predict as well as those features that will be used to make that prediction.
I can collect data on the down time of every piece of equipment on my factory floor and provide a daily snapshot, but unless I also have historical data on utilization and maintenance, no amount of analysis is going give me reliable predictions as to which machine will go down when.
This issue is one of data completeness. To link what you can see now to what you want to predict about the future, you need to have seen the data for both sides of the equation.
The transition to advisory systems, however, is somewhat different. In particular, it is different in that knowing what has happened and what might happen next is an exercise in understanding the world through the lens of data. Knowing what sort of advice to give you also requires understanding your business and your business goals.
Let me try to explain this with a ridiculous example.
Imagine that you have a commercial relationship with Bob. Bob, as it turns out, has tremendous arbitrage skills in the area of fruit. In particular, through a series of trades, Bob can reliably double the number of apples he has on a daily basis.
One aspect of your relationship with Bob is that you have an agreement in place that means you can take half of his apples from him on either a daily or weekly basis. The only directive is that once you decide on the cycle, you have to keep to it.
Now, if you choose once a day, you gain an apple every day and Bob remains at a steady state with no growth at all. Every time he gets a new apple, you take it away from him.
On the other hand, if you take half of Bob’s apples on a weekly basis, you both participate in an exponential growth of apples from this point on. Bob’s apple total doubles every day and yours every week.
As you look at this from a numbers or optimization point of view, the advice seems obvious: take half of Bob’s apples each week and you both end up with a lot of apples. In general, this is what people on the quant side tend to see.
This sounds great until you start asking more in-depth questions. Do you have a warehouse for all of these apples? Do you have a distribution network to sell them? Do you have a cider or apple sauce business that you want to grow? Do you have a partnership with a pie company that can use your now expanding apple supply?
Do you have a need for or a desire to have all these apples?
Maybe you don’t want all those apples. Maybe you simply embraced the notion that an apple a day keeps the doctor away and your apple needs are determined by personal health goals.
In short, what are your business goals with regard to Bob and his apples?
The point here is simple. In order for a system to go beyond the ability to assess and predict to then advise, your business goals need to literally be part of the equation. The first two components of analysis are aimed at drawing out information about the world from the data at hand. The final component, the ability to advise, is linked to information about your business goals. The trick is to make sure that you keep those goals in mind as you consider the nature of any advisory system.
Optimization systems that are focused on metrics such as machine utilization, route planning and inventory management are able to support advisory functions because the goals are clear and often linked to the same data that is used to provide assessment and prediction. Having clear business goals makes it possible for these systems to not just advise but often control systems to optimize.
But as the business goals become more fluid, driven by context, or outside of the data flow, the ability of systems to provide advice becomes more difficult to support.
In considering customer retention, for example, transactional data can often be used to learn the conditions that are predictive of churn. But the issue of how to respond, which requires historical data on steps that were taken and outcomes, might not yet be available. Likewise, exogenous features (e.g. is this one of the last customers on a legacy product) that could provide greater context to shape advice might not be part of the dataset. In these cases, the advice provided by an analytics layer will end up being more generic or based on a specific set of assumptions.
The cautionary moment here is not that analytically supported advisory systems should be avoided. On the contrary, they are powerful tools for many aspects of your business. The takeaway is that advisory systems are based on a combination of an analysis of the world and some rather deep assumptions about your business goals. But those assumptions are exactly that, assumptions. If you don’t own those assumptions, you will get advice that is based on someone else’s notion of what you want. The result will be outcomes such as optimizing against short-term metrics like profit per customer when you actually want to optimize against the longer-term goal of exceptional customer service. The technology worked great, but the system didn’t understand what you wanted.
We sometimes respond to the results of analysis as though we are looking at ground truth. As you look at the output of anything that provides advice, however, you need to make sure that your business goals are part of that truth. If you don’t, you will be using great technology that is doing a good job of giving you bad advice.
So, the next time a machine provides you with advice, ask yourself if it thinks that you want to go into the apple business.